FEATURED
Tariff Tremors, Market Rotations, and the Imperative of Optimization
The first quarter of 2025 marked a significant departure from the preceding two years, which had been characterized by an improving global economy and correspondingly positive market returns. Market performance in Q1 was dominated by abrupt, short-term policy shifts rather than longer-term economic trends, and tariffs became the foremost concern for market participants.
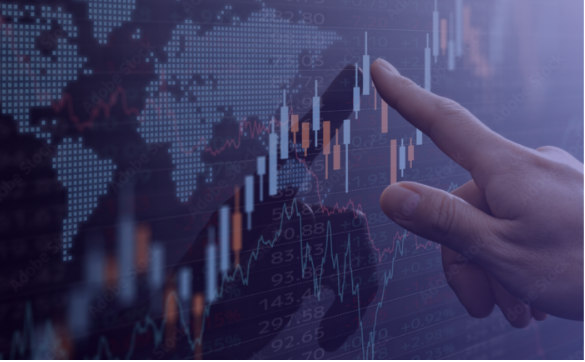